-
Discovering Low-Dimensional Descriptions of Multineuronal Dependencies
-
Differential Shannon Entropies Characterizing Electron–Nuclear Dynamics and Correlation: Momentum-Space Versus Coordinate-Space Wave Packet Motion
-
Bandit Algorithm Driven by a Classical Random Walk and a Quantum Walk
-
Psychological Implicit Motives Construct as an Emergent Fractal Attractor from Intermittent Neurophysiological Responses: Simulation and Entropy-like Characterization
-
Pattern Formation under Deep Supercooling by Classical Density Functional-Based Approach
Journal Description
Entropy
Entropy
is an international and interdisciplinary peer-reviewed open access journal of entropy and information studies, published monthly online by MDPI. The International Society for the Study of Information (IS4SI) and Spanish Society of Biomedical Engineering (SEIB) are affiliated with Entropy and their members receive a discount on the article processing charge.
- Open Access— free for readers, with article processing charges (APC) paid by authors or their institutions.
- High Visibility: indexed within Scopus, SCIE (Web of Science), MathSciNet, Inspec, PubMed, PMC, Astrophysics Data System, and other databases.
- Journal Rank: JCR - Q2 (Physics, Multidisciplinary) / CiteScore - Q1 (Mathematical Physics)
- Rapid Publication: manuscripts are peer-reviewed and a first decision is provided to authors approximately 20.4 days after submission; acceptance to publication is undertaken in 2.9 days (median values for papers published in this journal in the first half of 2023).
- Recognition of Reviewers: reviewers who provide timely, thorough peer-review reports receive vouchers entitling them to a discount on the APC of their next publication in any MDPI journal, in appreciation of the work done.
- Testimonials: See what our editors and authors say about Entropy.
- Companion journals for Entropy include: Foundations, Thermo and MAKE.
Impact Factor:
2.7 (2022);
5-Year Impact Factor:
2.6 (2022)
Latest Articles
Overview of Tensor-Based Cooperative MIMO Communication Systems—Part 1: Tensor Modeling
Entropy 2023, 25(8), 1181; https://doi.org/10.3390/e25081181 - 08 Aug 2023
Abstract
Due to increasingly strong and varied performance requirements, cooperative wireless communication systems today occupy a prominent place in both academic research and industrial development. The technological and economic challenges for future sixth-generation (6G) wireless systems are considerable, with the objectives of improving coverage,
[...] Read more.
Due to increasingly strong and varied performance requirements, cooperative wireless communication systems today occupy a prominent place in both academic research and industrial development. The technological and economic challenges for future sixth-generation (6G) wireless systems are considerable, with the objectives of improving coverage, data rate, latency, reliability, mobile connectivity and energy efficiency. Over the past decade, new technologies have emerged, such as massive multiple-input multiple-output (MIMO) relay systems, intelligent reflecting surfaces (IRS), unmanned aerial vehicular (UAV)-assisted communications, dual-polarized (DP) antenna arrays, three dimensional (3D) polarized channel modeling, and millimeter-wave (mmW) communication.
Full article
(This article belongs to the Special Issue Wireless Networks: Information Theoretic Perspectives III)
Open AccessFeature PaperArticle
Capacity-Achieving Input Distributions of Additive Vector Gaussian Noise Channels: Even-Moment Constraints and Unbounded or Compact Support
Entropy 2023, 25(8), 1180; https://doi.org/10.3390/e25081180 - 08 Aug 2023
Abstract
We investigate the support of a capacity-achieving input to a vector-valued Gaussian noise channel. The input is subjected to a radial even-moment constraint and is either allowed to take any value in or is restricted to a given compact subset of
[...] Read more.
We investigate the support of a capacity-achieving input to a vector-valued Gaussian noise channel. The input is subjected to a radial even-moment constraint and is either allowed to take any value in or is restricted to a given compact subset of . It is shown that the support of the capacity-achieving distribution is composed of a countable union of submanifolds, each with a dimension of or less. When the input is restricted to a compact subset of , this union is finite. Finally, the support of the capacity-achieving distribution is shown to have Lebesgue measure 0 and to be nowhere dense in .
Full article
(This article belongs to the Special Issue Shannon Entropy: Mathematical View)
Open AccessArticle
A Transverse Hamiltonian Approach to Infinitesimal Perturbation Analysis of Quantum Stochastic Systems
Entropy 2023, 25(8), 1179; https://doi.org/10.3390/e25081179 - 08 Aug 2023
Abstract
This paper is concerned with variational methods for open quantum systems with Markovian dynamics governed by Hudson–Parthasarathy quantum stochastic differential equations. These QSDEs are driven by quantum Wiener processes of the external bosonic fields and are specified by the system Hamiltonian and system–field
[...] Read more.
This paper is concerned with variational methods for open quantum systems with Markovian dynamics governed by Hudson–Parthasarathy quantum stochastic differential equations. These QSDEs are driven by quantum Wiener processes of the external bosonic fields and are specified by the system Hamiltonian and system–field coupling operators. We consider the system response to perturbations in these operators and introduce a transverse Hamiltonian which encodes the propagation of the perturbations through the unitary system–field evolution. This approach provides an infinitesimal perturbation analysis tool which can be used for the development of optimality conditions in quantum control and filtering problems. As performance criteria, such settings employ quadratic (or more complicated) cost functionals of the system and field variables to be minimized over the energy and coupling parameters of system interconnections. We demonstrate an application of the transverse Hamiltonian variational approach to a mean square optimal coherent quantum filtering problem for a measurement-free field-mediated cascade connection of a quantum system with a quantum observer.
Full article
(This article belongs to the Special Issue Quantum Probability and Randomness IV)
►▼
Show Figures
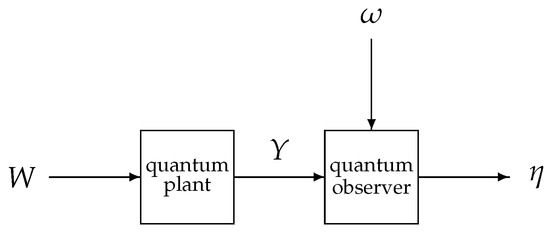
Figure 1
Open AccessArticle
An Image Encryption Algorithm Based on Improved Hilbert Curve Scrambling and Dynamic DNA Coding
Entropy 2023, 25(8), 1178; https://doi.org/10.3390/e25081178 - 08 Aug 2023
Abstract
As an effective method for image security protection, image encryption is widely used in data hiding and content protection. This paper proposes an image encryption algorithm based on an improved Hilbert curve with DNA coding. Firstly, the discrete wavelet transform (DWT) decomposes the
[...] Read more.
As an effective method for image security protection, image encryption is widely used in data hiding and content protection. This paper proposes an image encryption algorithm based on an improved Hilbert curve with DNA coding. Firstly, the discrete wavelet transform (DWT) decomposes the plaintext image by three-level DWT to obtain the high-frequency and low-frequency components. Secondly, different modes of the Hilbert curve are selected to scramble the high-frequency and low-frequency components. Then, the high-frequency and low-frequency components are reconstructed separately using the inverse discrete wavelet transform (IDWT). Then, the bit matrix of the image pixels is scrambled, changing the pixel value while changing the pixel position and weakening the strong correlation between adjacent pixels to a more significant correlation. Finally, combining dynamic DNA coding and ciphertext feedback to diffuse the pixel values improves the encryption effect. The encryption algorithm performs the scrambling and diffusion in alternating transformations of space, frequency, and spatial domains, breaking the limitations of conventional scrambling. The experimental simulation results and security analysis show that the encryption algorithm can effectively resist statistical attacks and differential attacks with good security and robustness.
Full article
(This article belongs to the Special Issue Image Encryption and Privacy Protection Based on Chaotic Systems)
►▼
Show Figures
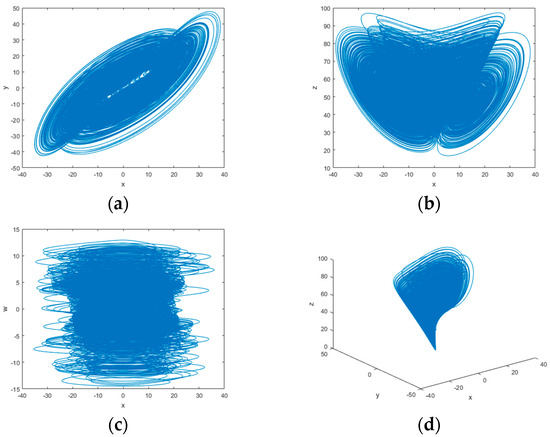
Figure 1
Open AccessCommunication
Geometric Insights into the Multivariate Gaussian Distribution and Its Entropy and Mutual Information
Entropy 2023, 25(8), 1177; https://doi.org/10.3390/e25081177 - 07 Aug 2023
Abstract
In this paper, we provide geometric insights with visualization into the multivariate Gaussian distribution and its entropy and mutual information. In order to develop the multivariate Gaussian distribution with entropy and mutual information, several significant methodologies are presented through the discussion, supported by
[...] Read more.
In this paper, we provide geometric insights with visualization into the multivariate Gaussian distribution and its entropy and mutual information. In order to develop the multivariate Gaussian distribution with entropy and mutual information, several significant methodologies are presented through the discussion, supported by illustrations, both technically and statistically. The paper examines broad measurements of structure for the Gaussian distributions, which show that they can be described in terms of the information theory between the given covariance matrix and correlated random variables (in terms of relative entropy). The content obtained allows readers to better perceive concepts, comprehend techniques, and properly execute software programs for future study on the topic’s science and implementations. It also helps readers grasp the themes’ fundamental concepts to study the application of multivariate sets of data in Gaussian distribution. The simulation results also convey the behavior of different elliptical interpretations based on the multivariate Gaussian distribution with entropy for real-world applications in our daily lives, including information coding, nonlinear signal detection, etc. Involving the relative entropy and mutual information as well as the potential correlated covariance analysis, a wide range of information is addressed, including basic application concerns as well as clinical diagnostics to detect the multi-disease effects.
Full article
(This article belongs to the Special Issue Entropy and Organization in Natural and Social Systems)
►▼
Show Figures
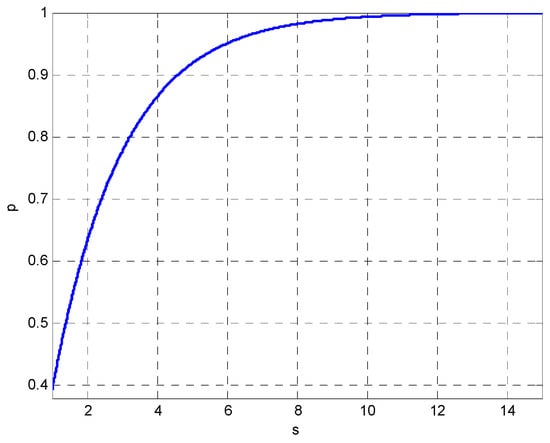
Figure 1
Open AccessArticle
Enhancing Image Quality via Robust Noise Filtering Using Redescending M-Estimators
by
, , and
Entropy 2023, 25(8), 1176; https://doi.org/10.3390/e25081176 - 07 Aug 2023
Abstract
In the field of image processing, noise represents an unwanted component that can occur during signal acquisition, transmission, and storage. In this paper, we introduce an efficient method that incorporates redescending M-estimators within the framework of Wiener estimation. The proposed approach effectively suppresses
[...] Read more.
In the field of image processing, noise represents an unwanted component that can occur during signal acquisition, transmission, and storage. In this paper, we introduce an efficient method that incorporates redescending M-estimators within the framework of Wiener estimation. The proposed approach effectively suppresses impulsive, additive, and multiplicative noise across varied densities. Our proposed filter operates on both grayscale and color images; it uses local information obtained from the Wiener filter and robust outlier rejection based on Insha and Hampel’s tripartite redescending influence functions. The effectiveness of the proposed method is verified through qualitative and quantitative results, using metrics such as PSNR, MAE, and SSIM.
Full article
(This article belongs to the Special Issue Pattern Recognition and Data Clustering in Information Theory)
►▼
Show Figures
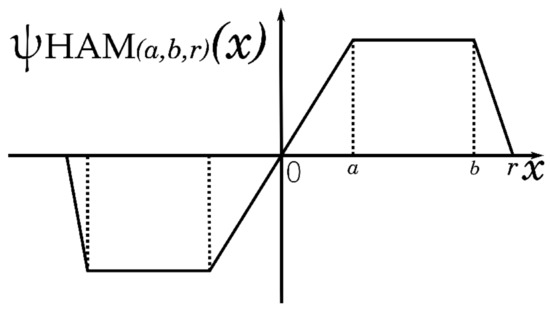
Figure 1
Open AccessArticle
Ensemble Improved Permutation Entropy: A New Approach for Time Series Analysis
Entropy 2023, 25(8), 1175; https://doi.org/10.3390/e25081175 - 07 Aug 2023
Abstract
Entropy quantification approaches have gained considerable attention in engineering applications. However, certain limitations persist, including the strong dependence on parameter selection, limited discriminating power, and low robustness to noise. To alleviate these issues, this paper introduces two novel algorithms for time series analysis:
[...] Read more.
Entropy quantification approaches have gained considerable attention in engineering applications. However, certain limitations persist, including the strong dependence on parameter selection, limited discriminating power, and low robustness to noise. To alleviate these issues, this paper introduces two novel algorithms for time series analysis: the ensemble improved permutation entropy (EIPE) and multiscale EIPE (MEIPE). Our approaches employ a new symbolization process that considers both permutation relations and amplitude information. Additionally, the ensemble technique is utilized to reduce the dependence on parameter selection. We performed a comprehensive evaluation of the proposed methods using various synthetic and experimental signals. The results illustrate that EIPE is capable of distinguishing white, pink, and brown noise with a smaller number of samples compared to traditional entropy algorithms. Furthermore, EIPE displays the potential to discriminate between regular and non-regular dynamics. Notably, when compared to permutation entropy, weighted permutation entropy, and dispersion entropy, EIPE exhibits superior robustness against noise. In practical applications, such as RR interval data classification, bearing fault diagnosis, marine vessel identification, and electroencephalographic (EEG) signal classification, the proposed methods demonstrate better discriminating power compared to conventional entropy measures. These promising findings validate the effectiveness and potential of the algorithms proposed in this paper.
Full article
(This article belongs to the Special Issue Information Theory and Nonlinear Signal Processing)
►▼
Show Figures
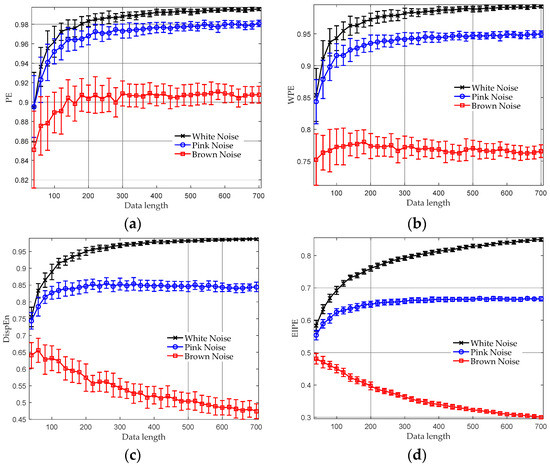
Figure 1
Open AccessArticle
Asymmetric Measurement-Device-Independent Quantum Key Distribution through Advantage Distillation
Entropy 2023, 25(8), 1174; https://doi.org/10.3390/e25081174 - 07 Aug 2023
Abstract
Measurement-device-independent quantum key distribution (MDI-QKD) completely closes the security loopholes caused by the imperfection of devices at the detection terminal. Commonly, a symmetric MDI-QKD model is widely used in simulations and experiments. This scenario is far from a real quantum network, where the
[...] Read more.
Measurement-device-independent quantum key distribution (MDI-QKD) completely closes the security loopholes caused by the imperfection of devices at the detection terminal. Commonly, a symmetric MDI-QKD model is widely used in simulations and experiments. This scenario is far from a real quantum network, where the losses of channels connecting each user are quite different. To adapt such a feature, an asymmetric MDI-QKD model is proposed. How to improve the performance of asymmetric MDI-QKD also becomes an important research direction. In this work, an advantage distillation (AD) method is applied to further improve the performance of asymmetric MDI-QKD without changing the original system structure. Simulation results show that the AD method can improve the secret key rate and transmission distance, especially in the highly asymmetric cases. Therefore, this scheme will greatly promote the development of future MDI-QKD networks.
Full article
(This article belongs to the Special Issue Advances in Quantum Computing)
►▼
Show Figures
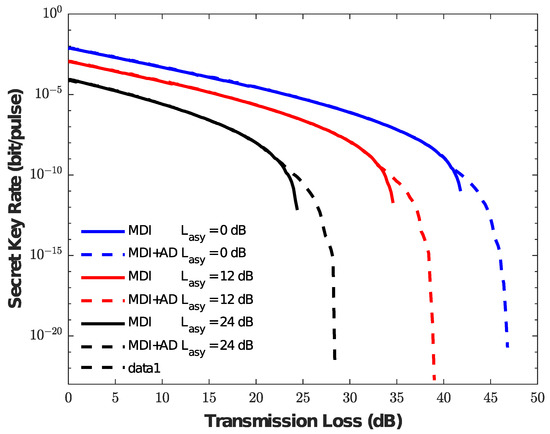
Figure 1
Open AccessArticle
Semiosis as a Source of Providing Empirical Phenomena with a New Type of Cohesion
Entropy 2023, 25(8), 1173; https://doi.org/10.3390/e25081173 - 07 Aug 2023
Abstract
Embodying the indexical signs is vital to semiosis as a cohesive material agency mediating between consequents and antecedents. One unique factor of biology compared with standard physics and chemistry is the cohesion enabling the biological components, codes and organizations to accommodate themselves with
[...] Read more.
Embodying the indexical signs is vital to semiosis as a cohesive material agency mediating between consequents and antecedents. One unique factor of biology compared with standard physics and chemistry is the cohesion enabling the biological components, codes and organizations to accommodate themselves with a specific material embodiment. Every individual body is uniquely biological and requires a specific cohesion of material origin for its own sake that could not be found in the non-living material world. The relevant cohesion comes from the exchange interaction of the atomic quantum particles, such as the carbon atoms, which is far greater than the electrons as a common exchange mediator adopted for the spatial cohesion ubiquitous in physics and chemistry. What is specific to the temporal cohesion latent in the atomic exchange is the immutable identity of the individual quantum particle surviving only over a limited time, while being constantly alternated with the new ones of the same kinds in a successive manner. Semiosis is supported by the underlying teleonomic cohesion, such that the preceding temporal cohesion may constantly induce the succeeding similar one ad infinitum.
Full article
(This article belongs to the Special Issue Probability, Entropy, Information, and Semiosis in Living Systems)
Open AccessArticle
Outage Performance Analysis of Improper Gaussian Signaling for Two-User Downlink NOMA Systems with Imperfect Successive Interference Cancellation
Entropy 2023, 25(8), 1172; https://doi.org/10.3390/e25081172 - 06 Aug 2023
Abstract
The improper Gaussian signaling (IGS) technique can improve the achievable rate of an interference-limited network by fully exploiting the second-order statistics of complex signaling. This paper addresses the outage performance analysis of a two-user downlink non-orthogonal multiple access (NOMA) system using the IGS
[...] Read more.
The improper Gaussian signaling (IGS) technique can improve the achievable rate of an interference-limited network by fully exploiting the second-order statistics of complex signaling. This paper addresses the outage performance analysis of a two-user downlink non-orthogonal multiple access (NOMA) system using the IGS technique in the presence of imperfect successive interference cancellation (SIC). The strong channel user (SU) adopts the IGS, while the weak channel user (WU) adopts the traditional proper Gaussian signaling (PGS). Considering a practical scenario where the transmitter has obtained the statistics of the channel coefficients instead of the instantaneous channel state information (CSI), the expressions of the achievable rates of both users under residual interference due to imperfect SIC are derived, together with their outage probabilities, subject to predetermined target rates and channel statistics. Given a fixed transmit power of the WU, both the transmit power and the degree of impropriety of the SU are optimized to minimize the outage probability subject to the outage constraint of the WU. Numerical results are provided to assess the benefits of the proposed IGS-based downlink NOMA system, which are consistent with the analysis.
Full article
(This article belongs to the Section Information Theory, Probability and Statistics)
►▼
Show Figures
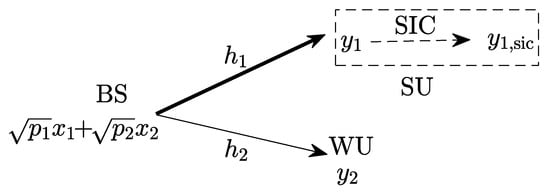
Figure 1
Open AccessArticle
Improved Adaptive Multipoint Optimal Minimum Entropy Deconvolution and Application on Bearing Fault Detection in Random Impulsive Noise Environments
Entropy 2023, 25(8), 1171; https://doi.org/10.3390/e25081171 - 06 Aug 2023
Abstract
Random impulsive noise is a special kind of noise, which has strong impact features and random disturbances with large amplitude, short duration, and long intervals. This type of noise often displays nonGaussianity, while common background noise obeys Gaussian distribution. Hence, random impulsive noise
[...] Read more.
Random impulsive noise is a special kind of noise, which has strong impact features and random disturbances with large amplitude, short duration, and long intervals. This type of noise often displays nonGaussianity, while common background noise obeys Gaussian distribution. Hence, random impulsive noise greatly differs from common background noise, which renders many commonly used approaches in bearing fault diagnosis inapplicable. In this work, we explore the challenge of bearing fault detection in the presence of random impulsive noise. To deal with this issue, an improved adaptive multipoint optimal minimum entropy deconvolution (IAMOMED) is introduced. In this IAMOMED, an envelope autocorrelation function is used to automatically estimate the cyclic impulse period instead of setting an approximate period range. Moreover, the target vector in the original MOMED is rearranged to enhance its practical applicability. Finally, particle swarm optimization is employed to determine the optimal filter length for selection purposes. According to these improvements, IAMOMED is more suitable for detecting bearing fault features in the case of random impulsive noise when compared to the original MOMED. The contrast experiments demonstrate that the proposed IAMOMED technique is capable of effectively identifying fault characteristics from the vibration signal with strong random impulsive noise and, in addition, it can accurately diagnose the fault types. Thus, the proposed method provides an alternative fault detection tool for rotating machinery in the presence of random impulsive noise.
Full article
(This article belongs to the Section Multidisciplinary Applications)
►▼
Show Figures
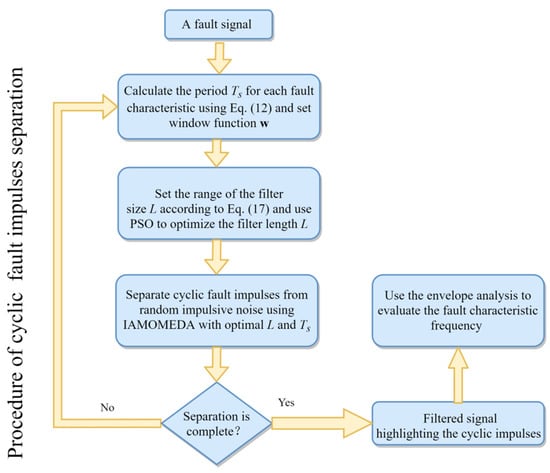
Figure 1
Open AccessArticle
Identification of Solid and Liquid Materials Using Acoustic Signals and Frequency-Graph Features
by
and
Entropy 2023, 25(8), 1170; https://doi.org/10.3390/e25081170 - 05 Aug 2023
Abstract
►▼
Show Figures
Material identification is playing an increasingly important role in various sectors such as industry, petrochemical, mining, and in our daily lives. In recent years, material identification has been utilized for security checks, waste sorting, etc. However, current methods for identifying materials require direct
[...] Read more.
Material identification is playing an increasingly important role in various sectors such as industry, petrochemical, mining, and in our daily lives. In recent years, material identification has been utilized for security checks, waste sorting, etc. However, current methods for identifying materials require direct contact with the target and specialized equipment that can be costly, bulky, and not easily portable. Past proposals for addressing this limitation relied on non-contact material identification methods, such as Wi-Fi-based and radar-based material identification methods, which can identify materials with high accuracy without physical contact; however, they are not easily integrated into portable devices. This paper introduces a novel non-contact material identification based on acoustic signals. Different from previous work, our design leverages the built-in microphone and speaker of smartphones as the transceiver to identify target materials. The fundamental idea of our design is that acoustic signals, when propagated through different materials, reach the receiver via multiple paths, producing distinct multipath profiles. These profiles can serve as fingerprints for material identification. We captured and extracted them using acoustic signals, calculated channel impulse response (CIR) measurements, and then extracted image features from the time–frequency domain feature graphs, including histogram of oriented gradient (HOG) and gray-level co-occurrence matrix (GLCM) image features. Furthermore, we adopted the error-correcting output code (ECOC) learning method combined with the majority voting method to identify target materials. We built a prototype for this paper using three mobile phones based on the Android platform. The results from three different solid and liquid materials in varied multipath environments reveal that our design can achieve average identification accuracies of 90% and 97%.
Full article
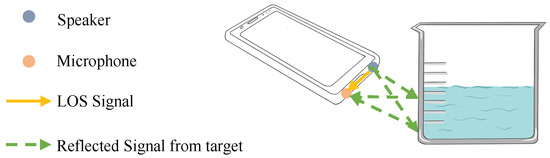
Figure 1
Open AccessArticle
Distance Matters: A Distance-Aware Medical Image Segmentation Algorithm
Entropy 2023, 25(8), 1169; https://doi.org/10.3390/e25081169 - 05 Aug 2023
Abstract
The transformer-based U-Net network structure has gained popularity in the field of medical image segmentation. However, most networks overlook the impact of the distance between each patch on the encoding process. This paper proposes a novel GC-TransUnet for medical image segmentation. The key
[...] Read more.
The transformer-based U-Net network structure has gained popularity in the field of medical image segmentation. However, most networks overlook the impact of the distance between each patch on the encoding process. This paper proposes a novel GC-TransUnet for medical image segmentation. The key innovation is that it takes into account the relationships between patch blocks based on their distances, optimizing the encoding process in traditional transformer networks. This optimization results in improved encoding efficiency and reduced computational costs. Moreover, the proposed GC-TransUnet is combined with U-Net to accomplish the segmentation task. In the encoder part, the traditional vision transformer is replaced by the global context vision transformer (GC-VIT), eliminating the need for the CNN network while retaining skip connections for subsequent decoders. Experimental results demonstrate that the proposed algorithm achieves superior segmentation results compared to other algorithms when applied to medical images.
Full article
(This article belongs to the Special Issue Deep Learning Models and Applications to Computer Vision)
►▼
Show Figures
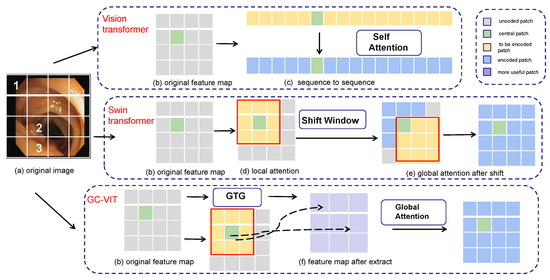
Figure 1
Open AccessArticle
Quantifying Parameter Interdependence in Stochastic Discrete Models of Biochemical Systems
by
and
Entropy 2023, 25(8), 1168; https://doi.org/10.3390/e25081168 - 05 Aug 2023
Abstract
Stochastic modeling of biochemical processes at the cellular level has been the subject of intense research in recent years. The Chemical Master Equation is a broadly utilized stochastic discrete model of such processes. Numerous important biochemical systems consist of many species subject to
[...] Read more.
Stochastic modeling of biochemical processes at the cellular level has been the subject of intense research in recent years. The Chemical Master Equation is a broadly utilized stochastic discrete model of such processes. Numerous important biochemical systems consist of many species subject to many reactions. As a result, their mathematical models depend on many parameters. In applications, some of the model parameters may be unknown, so their values need to be estimated from the experimental data. However, the problem of parameter value inference can be quite challenging, especially in the stochastic setting. To estimate accurately the values of a subset of parameters, the system should be sensitive with respect to variations in each of these parameters and they should not be correlated. In this paper, we propose a technique for detecting collinearity among models’ parameters and we apply this method for selecting subsets of parameters that can be estimated from the available data. The analysis relies on finite-difference sensitivity estimations and the singular value decomposition of the sensitivity matrix. We illustrated the advantages of the proposed method by successfully testing it on several models of biochemical systems of practical interest.
Full article
(This article belongs to the Special Issue Mathematical Modeling in Systems Biology)
►▼
Show Figures
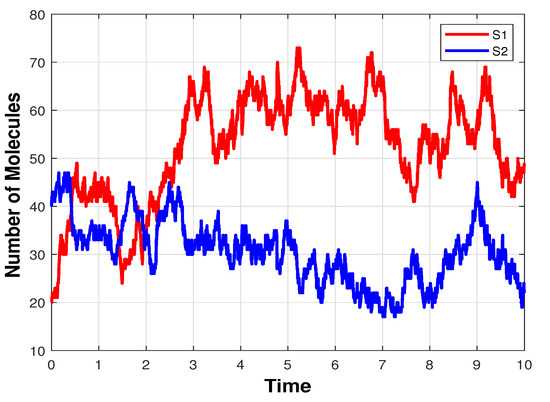
Figure 1
Open AccessArticle
NRVC: Neural Representation for Video Compression with Implicit Multiscale Fusion Network
Entropy 2023, 25(8), 1167; https://doi.org/10.3390/e25081167 - 04 Aug 2023
Abstract
Recently, end-to-end deep models for video compression have made steady advancements. However, this resulted in a lengthy and complex pipeline containing numerous redundant parameters. The video compression approaches based on implicit neural representation (INR) allow videos to be directly represented as a function
[...] Read more.
Recently, end-to-end deep models for video compression have made steady advancements. However, this resulted in a lengthy and complex pipeline containing numerous redundant parameters. The video compression approaches based on implicit neural representation (INR) allow videos to be directly represented as a function approximated by a neural network, resulting in a more lightweight model, whereas the singularity of the feature extraction pipeline limits the network’s ability to fit the mapping function for video frames. Hence, we propose a neural representation approach for video compression with an implicit multiscale fusion network (NRVC), utilizing normalized residual networks to improve the effectiveness of INR in fitting the target function. We propose the multiscale representations for video compression (MSRVC) network, which effectively extracts features from the input video sequence to enhance the degree of overfitting in the mapping function. Additionally, we propose the feature extraction channel attention (FECA) block to capture interaction information between different feature extraction channels, further improving the effectiveness of feature extraction. The results show that compared to the NeRV method with similar bits per pixel (BPP), NRVC has a 2.16% increase in the decoded peak signal-to-noise ratio (PSNR). Moreover, NRVC outperforms the conventional HEVC in terms of PSNR.
Full article
(This article belongs to the Topic Recent Trends in Image Processing and Pattern Recognition)
►▼
Show Figures
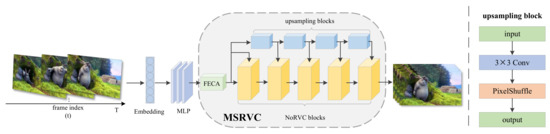
Figure 1
Open AccessArticle
Task-Decoupled Knowledge Transfer for Cross-Modality Object Detection
Entropy 2023, 25(8), 1166; https://doi.org/10.3390/e25081166 - 04 Aug 2023
Abstract
In harsh weather conditions, the infrared modality can supplement or even replace the visible modality. However, the lack of a large-scale dataset for infrared features hinders the generation of a robust pre-training model. Most existing infrared object-detection algorithms rely on pre-training models from
[...] Read more.
In harsh weather conditions, the infrared modality can supplement or even replace the visible modality. However, the lack of a large-scale dataset for infrared features hinders the generation of a robust pre-training model. Most existing infrared object-detection algorithms rely on pre-training models from the visible modality, which can accelerate network convergence but also limit performance due to modality differences. In order to provide more reliable feature representation for cross-modality object detection and enhance its performance, this paper investigates the impact of various task-relevant features on cross-modality object detection and proposes a knowledge transfer algorithm based on classification and localization decoupling analysis. A task-decoupled pre-training method is introduced to adjust the attributes of various tasks learned by the pre-training model. For the training phase, a task-relevant hyperparameter evolution method is proposed to increase the network’s adaptability to attribute changes in pre-training weights. Our proposed method improves the accuracy of multiple modalities in multiple datasets, with experimental results on the FLIR ADAS dataset reaching a state-of-the-art level and surpassing most multi-spectral object-detection methods.
Full article
(This article belongs to the Special Issue Machine and Deep Learning for Affective Computing)
►▼
Show Figures
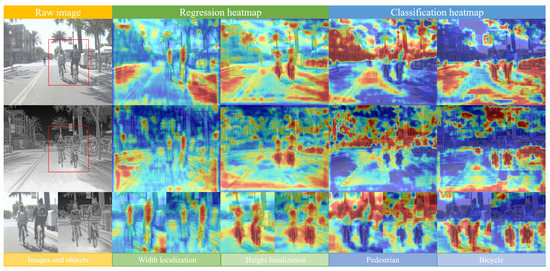
Figure 1
Open AccessArticle
A Multiscale Recursive Attention Gate Federation Method for Multiple Working Conditions Fault Diagnosis
Entropy 2023, 25(8), 1165; https://doi.org/10.3390/e25081165 - 04 Aug 2023
Abstract
Federated learning (FL) is an effective method when a single client cannot provide enough samples for multiple condition fault diagnosis of bearings since it can combine the information provided by multiple clients. However, some of the client’s working conditions are different; for example,
[...] Read more.
Federated learning (FL) is an effective method when a single client cannot provide enough samples for multiple condition fault diagnosis of bearings since it can combine the information provided by multiple clients. However, some of the client’s working conditions are different; for example, different clients are in different stages of the whole life cycle, and different clients have different loads. At this point, the status of each client is not equal, and the traditional FL approach will lead to some clients’ useful information being ignored. The purpose of this paper is to investigate a multiscale recursive FL framework that makes the server more focused on the useful information provided by the clients to ensure the effectiveness of FL. The proposed FL method can build reliable multiple working condition fault diagnosis models due to the increased focus on useful information in the FL process and the full utilization of server information through local multiscale feature fusion. The validity of the proposed method was verified with the Case Western Reserve University benchmark dataset. With less local client training data and complex fault types, the proposed method improves the accuracy of fault diagnosis by 23.21% over the existing FL fault diagnosis.
Full article
(This article belongs to the Special Issue New Trends in Fault Diagnosis and Prognosis for Engineering Applications: From Signal Processing to Machine Learning and Deep Learning)
►▼
Show Figures
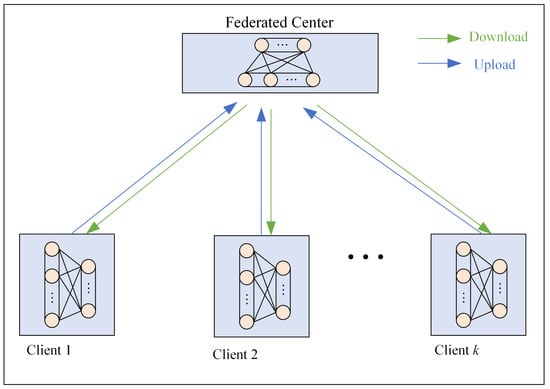
Figure 1
Open AccessArticle
Causal Responsibility Division of Chronological Continuous Treatment Based on Change-Point Detection
Entropy 2023, 25(8), 1164; https://doi.org/10.3390/e25081164 - 03 Aug 2023
Abstract
This paper introduces a novel approach, called causal relation quantification, based on change-point detection to address the issue of harmonic responsibility division in power systems. The proposed method focuses on determining the causal effect of chronological continuous treatment, enabling the identification of crucial
[...] Read more.
This paper introduces a novel approach, called causal relation quantification, based on change-point detection to address the issue of harmonic responsibility division in power systems. The proposed method focuses on determining the causal effect of chronological continuous treatment, enabling the identification of crucial treatment intervals. Within each interval, three propensity-score-based algorithms are executed to assess their respective causal effects. By integrating the results from each interval, the overall causal effect of a chronological continuous treatment variable can be calculated. This calculated overall causal effect represents the causal responsibility of each harmonic customer. The effectiveness of the proposed method is evaluated through a simulation study and demonstrated in an empirical harmonic application. The results of the simulation study indicate that our method provides accurate and robust estimates, while the calculated results in the harmonic application align closely with the real-world scenario as verified by on-site investigations.
Full article
(This article belongs to the Section Signal and Data Analysis)
►▼
Show Figures
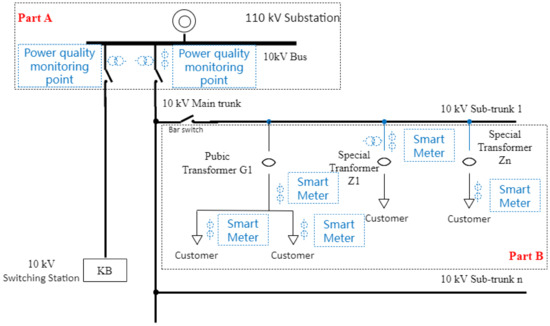
Figure 1
Open AccessArticle
Superimposed Perfect Binary Array-Aided Channel Estimation for OTFS Systems
Entropy 2023, 25(8), 1163; https://doi.org/10.3390/e25081163 - 03 Aug 2023
Abstract
Orthogonal time-frequency space (OTFS) modulation outperforms orthogonal frequency-division multiplexing in high-mobility scenarios through better channel estimation. Current superimposed pilot (SP)-based channel estimation improves the spectral efficiency (SE) when compared to that of the traditional embedded pilot (EP) method. However, it requires an additional
[...] Read more.
Orthogonal time-frequency space (OTFS) modulation outperforms orthogonal frequency-division multiplexing in high-mobility scenarios through better channel estimation. Current superimposed pilot (SP)-based channel estimation improves the spectral efficiency (SE) when compared to that of the traditional embedded pilot (EP) method. However, it requires an additional non-superimposed EP delay-Doppler frame to estimate the delay-Doppler taps for the following SP-aided frames. To handle this problem, we propose a channel estimation method with high SE, which superimposes the perfect binary array (PBA) on data symbols as the pilot. Utilizing the perfect autocorrelation of PBA, channel estimation is performed based on a linear search to find the correlation peaks, which include both delay-Doppler tap information and complex channel gain in the same superimposed PBA frame. Furthermore, the optimal power ratio of the PBA is then derived by maximizing the signal-to-interference-plus-noise ratio (SINR) to optimize the SE of the proposed system. The simulation results demonstrate that the proposed method can achieve a similar channel estimation performance to the existing EP method while significantly improving the SE.
Full article
(This article belongs to the Special Issue Delay-Doppler Domain Communications for Future Wireless Networks)
►▼
Show Figures
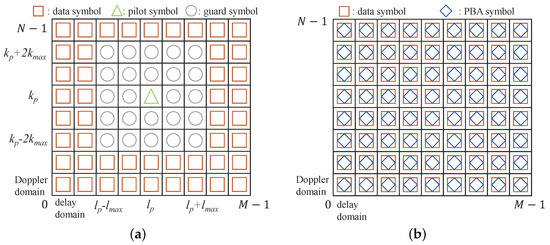
Figure 1
Open AccessArticle
Precise Traits from Sloppy Components: Perception and the Origin of Phenotypic Response
Entropy 2023, 25(8), 1162; https://doi.org/10.3390/e25081162 - 03 Aug 2023
Abstract
Organisms perceive their environment and respond. The origin of perception–response traits presents a puzzle. Perception provides no value without response. Response requires perception. Recent advances in machine learning may provide a solution. A randomly connected network creates a reservoir of perceptive information about
[...] Read more.
Organisms perceive their environment and respond. The origin of perception–response traits presents a puzzle. Perception provides no value without response. Response requires perception. Recent advances in machine learning may provide a solution. A randomly connected network creates a reservoir of perceptive information about the recent history of environmental states. In each time step, a relatively small number of inputs drives the dynamics of the relatively large network. Over time, the internal network states retain a memory of past inputs. To achieve a functional response to past states or to predict future states, a system must learn only how to match states of the reservoir to the target response. In the same way, a random biochemical or neural network of an organism can provide an initial perceptive basis. With a solution for one side of the two-step perception–response challenge, evolving an adaptive response may not be so difficult. Two broader themes emerge. First, organisms may often achieve precise traits from sloppy components. Second, evolutionary puzzles often follow the same outlines as the challenges of machine learning. In each case, the basic problem is how to learn, either by artificial computational methods or by natural selection.
Full article
(This article belongs to the Special Issue Mathematical Modeling in Systems Biology)
►▼
Show Figures
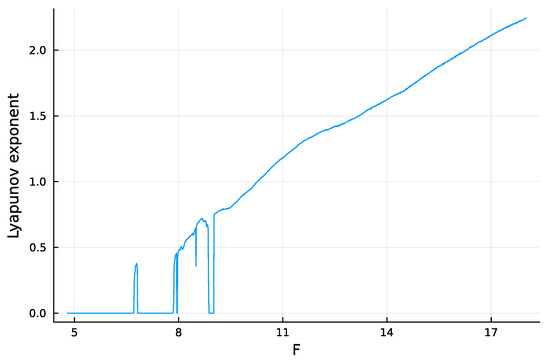
Figure 1
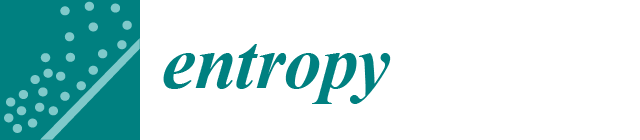
Journal Menu
► ▼ Journal Menu-
- Entropy Home
- Aims & Scope
- Editorial Board
- Reviewer Board
- Topical Advisory Panel
- Video Exhibition
- Instructions for Authors
- Special Issues
- Topics
- Sections & Collections
- Article Processing Charge
- Indexing & Archiving
- Editor’s Choice Articles
- Most Cited & Viewed
- Journal Statistics
- Journal History
- Journal Awards
- Society Collaborations
- Conferences
- Editorial Office
Journal Browser
► ▼ Journal Browser-
arrow_forward_ios
Forthcoming issue
arrow_forward_ios Current issue - Vol. 25 (2023)
- Vol. 24 (2022)
- Vol. 23 (2021)
- Vol. 22 (2020)
- Vol. 21 (2019)
- Vol. 20 (2018)
- Vol. 19 (2017)
- Vol. 18 (2016)
- Vol. 17 (2015)
- Vol. 16 (2014)
- Vol. 15 (2013)
- Vol. 14 (2012)
- Vol. 13 (2011)
- Vol. 12 (2010)
- Vol. 11 (2009)
- Vol. 10 (2008)
- Vol. 9 (2007)
- Vol. 8 (2006)
- Vol. 7 (2005)
- Vol. 6 (2004)
- Vol. 5 (2003)
- Vol. 4 (2002)
- Vol. 3 (2001)
- Vol. 2 (2000)
- Vol. 1 (1999)
Highly Accessed Articles
Latest Books
E-Mail Alert
News
Topics
Topic in
Energies, Mathematics, Entropy, Computers, Physics
Numerical Methods and Computer Simulations in Energy Analysis, 2nd Volume
Topic Editors: Marcin Kamiński, Mateus MendesDeadline: 31 August 2023
Topic in
Entropy, Algorithms, Computation, MAKE, Energies, Materials
Artificial Intelligence and Computational Methods: Modeling, Simulations and Optimization of Complex Systems
Topic Editors: Jaroslaw Krzywanski, Yunfei Gao, Marcin Sosnowski, Karolina Grabowska, Dorian Skrobek, Ghulam Moeen Uddin, Anna Kulakowska, Anna Zylka, Bachil El FilDeadline: 20 October 2023
Topic in
Coatings, Energies, Entropy, Foundations, Nanomaterials, Symmetry, Thermo
Heat Transfer Enhancement and Applications
Topic Editors: Mikhail Sheremet, Abderrahmane BaïriDeadline: 30 October 2023
Topic in
Energies, Entropy, Photonics, Technologies, Thermo
Advances in Solar Technologies
Topic Editors: Jayanta Deb Mondol, Annamaria Buonomano, Biplab DasDeadline: 10 November 2023

Conferences
Special Issues
Special Issue in
Entropy
Information Theory and 5G/6G Wireless Communication System
Guest Editor: Ke XiongDeadline: 10 August 2023
Special Issue in
Entropy
Entropy Based Data Hiding and Its Applications
Guest Editors: Tzu Chuen Lu, David MegíasDeadline: 15 August 2023
Special Issue in
Entropy
Applied Probability, Information Theory and Applications
Guest Editors: Dimitris Kugiumtzis, George TsaklidisDeadline: 31 August 2023
Special Issue in
Entropy
Optical Communication and Information Technology
Guest Editors: Mario Martinelli, Pierpaolo BoffiDeadline: 10 September 2023
Topical Collections
Topical Collection in
Entropy
Algorithmic Information Dynamics: A Computational Approach to Causality from Cells to Networks
Collection Editors: Hector Zenil, Felipe Abrahão
Topical Collection in
Entropy
Wavelets, Fractals and Information Theory
Collection Editor: Carlo Cattani
Topical Collection in
Entropy
Entropy in Image Analysis
Collection Editor: Amelia Carolina Sparavigna